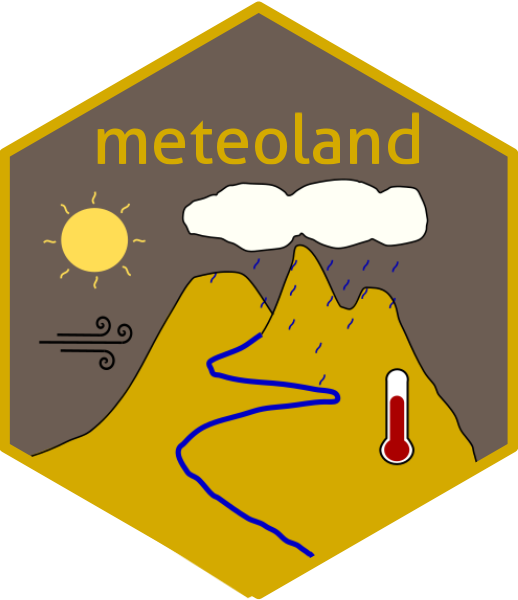
Summarise interpolated data by temporal dimension
Source:R/tidy_summary_interpolated_data.R
summarise_interpolated_data.Rd
Usage
summarise_interpolated_data(
interpolated_data,
fun = "mean",
frequency = NULL,
vars_to_summary = c("MeanTemperature", "MinTemperature", "MaxTemperature",
"Precipitation", "MeanRelativeHumidity", "MinRelativeHumidity",
"MaxRelativeHumidity", "Radiation", "WindSpeed", "WindDirection", "PET"),
dates_to_summary = NULL,
months_to_summary = 1:12,
verbose = getOption("meteoland_verbosity", TRUE),
...
)
Arguments
- interpolated_data
An interpolated data object as returned by
interpolate_data
.- fun
The function to use for summarising the data.
- frequency
A string indicating the interval specification (allowed ones are "week", "month", "quarter" and "year"). If NULL (default), aggregation is done in one interval for all the dates present.
- vars_to_summary
A character vector with one or more variable names to summarise. By default, all interpolated variables are summarised.
- dates_to_summary
A Date object to define the dates to be summarised. If NULL (default), all dates in the interpolated data are processed.
- months_to_summary
A numeric vector with the month numbers to subset the data before summarising. (e.g.
c(7,8)
for July and August). This parameter allows studying particular seasons, when combined withfrequency
. For examplefrequency = "years"
andmonths = 6:8
leads to summarizing summer months of each year.- verbose
Logical indicating if the function must show messages and info. Default value checks
"meteoland_verbosity"
option and if not set, defaults to TRUE. It can be turned off for the function with FALSE, or session wide withoptions(meteoland_verbosity = FALSE)
- ...
Arguments needed for
fun
Value
For a nested interpolated data, the same sf object with a new column with the temporal summaries. For an unnested interpolated data, a data.frame with the summarised meteo variables. For an interpolated raster (stars object), the same raster with the temporal dimension aggregated as desired.
Details
If interpolated_data
is a nested interpolated data sf object, as
returned by interpolate_data
, temporal summary is done for each
location present in the interpolated data. If interpolated_data
is
an unnested interpolated data sf object, temporal summary is done for all
locations together. If interpolated_data
is a single location data.frame
containing the dates and the interpolated variables, temporal summary is done
for that location. If interpolated_data
is a stars object as returned
by interpolate_data
, temporal summary is done for all the raster.
Examples
# \donttest{
# points interpolation aggregation
points_to_interpolate_example |>
interpolate_data(meteoland_interpolator_example, verbose = FALSE) |>
summarise_interpolated_data()
#> Simple feature collection with 15 features and 6 fields
#> Geometry type: POINT
#> Dimension: XY
#> Bounding box: xmin: 0.7578958 ymin: 41.31541 xmax: 2.98657 ymax: 42.6336
#> Geodetic CRS: WGS 84
#> # A tibble: 15 × 7
#> plot_id elevation slope aspect geometry interpolated_data
#> * <chr> <dbl> <dbl> <dbl> <POINT [°]> <list>
#> 1 P_05284 889. 25.2 313. (2.320167 42.24139) <tibble [30 × 13]>
#> 2 P_06572 680. 18.0 79.9 (2.552233 42.02596) <tibble [30 × 13]>
#> 3 P_07201 141. 4.17 52.9 (2.721874 41.88258) <tibble [30 × 13]>
#> 4 P_07512 254. 14.3 261. (2.98657 41.9006) <tibble [30 × 13]>
#> 5 P_08207 1860. 36.4 293. (2.209903 42.33968) <tibble [30 × 13]>
#> 6 P_08299 183. 4.12 92.9 (2.817143 42.24325) <tibble [30 × 13]>
#> 7 P_09341 819 23.4 128. (1.126766 42.42612) <tibble [30 × 13]>
#> 8 P_10272 860 34.8 347. (1.398528 42.26791) <tibble [30 × 13]>
#> 9 P_10861 706 22.4 22.6 (0.9314126 42.04226) <tibble [30 × 13]>
#> 10 P_11651 585 22.0 199. (0.7578958 41.8612) <tibble [30 × 13]>
#> 11 P_12150 674. 30.3 154. (1.481719 41.81838) <tibble [30 × 13]>
#> 12 P_12227 752. 6.04 27.7 (1.283161 41.591) <tibble [30 × 13]>
#> 13 P_12417 702 11.6 63.1 (0.8727224 41.35875) <tibble [30 × 13]>
#> 14 P_13007 972. 4.21 338. (1.120383 42.6336) <tibble [30 × 13]>
#> 15 P_14029 556. 14.1 41.4 (1.480716 41.31541) <tibble [30 × 13]>
#> # ℹ 1 more variable: all_mean <list>
# raster interpolation aggregation
raster_to_interpolate_example |>
interpolate_data(meteoland_interpolator_example, verbose = FALSE) |>
summarise_interpolated_data()
#> stars object with 3 dimensions and 11 attributes
#> attribute(s):
#> Min. 1st Qu. Median Mean 3rd Qu.
#> MeanTemperature 11.5704340 13.085384 13.580366 13.471788 14.000217
#> MinTemperature 5.4241252 5.723106 5.834485 5.860040 6.015028
#> MaxTemperature 15.4618660 17.809716 18.593723 18.420680 19.205037
#> Precipitation 1.0384680 1.131101 1.199751 1.217155 1.293790
#> MeanRelativeHumidity 60.7962965 63.361941 65.087755 65.520741 67.043155
#> MinRelativeHumidity 43.3452715 46.019400 48.149531 48.591400 50.195214
#> MaxRelativeHumidity 96.2942503 96.710474 96.899356 96.878881 97.036136
#> Radiation 13.9846077 18.365970 20.168242 19.595816 21.198293
#> WindSpeed 0.9485546 1.032881 1.262741 1.385929 1.680294
#> WindDirection NA NA NA NaN NA
#> PET 1.9918440 2.851873 3.153198 3.087621 3.402888
#> Max. NA's
#> MeanTemperature 14.659490 0
#> MinTemperature 6.280687 0
#> MaxTemperature 20.321977 0
#> Precipitation 1.445986 0
#> MeanRelativeHumidity 73.956489 0
#> MinRelativeHumidity 58.061458 0
#> MaxRelativeHumidity 97.482022 0
#> Radiation 22.812720 0
#> WindSpeed 2.382469 1
#> WindDirection NA 121
#> PET 4.214379 0
#> dimension(s):
#> from to offset delta refsys point values x/y
#> time 1 1 NA NA POSIXct NA 2022-04-01 CEST
#> x 1 11 1.671 0.01058 WGS 84 FALSE NULL [x]
#> y 1 11 41.76 -0.01058 WGS 84 FALSE NULL [y]
# }